Note
Click here to download the full example code
Example for evolutionary regression with multiple genomesΒΆ
Example demonstrating the use of Cartesian genetic programming with multiple genomes per individual for a simple regression task with a piecewise target function.
# The docopt str is added explicitly to ensure compatibility with
# sphinx-gallery.
docopt_str = """
Usage:
example_multi_genome.py [--max-generations=<N>]
Options:
-h --help
--max-generations=<N> Maximum number of generations [default: 300]
"""
import matplotlib.pyplot as plt
import numpy as np
import scipy.constants
from docopt import docopt
import cgp
args = docopt(docopt_str)
We first define a target function. The function applies different transformations to the input depending whether the input is less or greater than or equal to zero. We thus need to fit two different functions.
def f_target(x):
return np.select([x < 0, x >= 0], [-x, x ** 2 + 1.0])
Then we define the objective function for the evolution. It uses the
mean-squared error between the output of the expression represented by a given
individual and the target function evaluated on a set of random points. We
here either evaluate the function represented by the first (f[0]
) or the second
genome (f[1]
), depending whether the input is less or greater than zero.
def objective(individual):
if not individual.fitness_is_None():
return individual
n_function_evaluations = 1000
np.random.seed(1234)
# Note that f is now a list of functions because individual is an instance
# of `InvidividualMultiGenome`
f = individual.to_numpy()
x = np.random.uniform(-4, 4, n_function_evaluations)
y = np.piecewise(x, [x < 0, x >= 0], f)
loss = np.sum((f_target(x) - y) ** 2)
individual.fitness = -loss / n_function_evaluations
return individual
Next, we set up the evolutionary search. First, we define the parameters for
the population, the genomes of individuals, and the evolutionary
algorithm. Note that we define genome_params
as a list of parameter
dictionaries which causes the population to create instances of
InvidividualMultiGenome
.
population_params = {"n_parents": 1, "seed": 8188211}
single_genome_params = {
"n_inputs": 1,
"n_outputs": 1,
"n_columns": 12,
"n_rows": 1,
"levels_back": 5,
"primitives": (cgp.Add, cgp.Sub, cgp.Mul, cgp.ConstantFloat),
}
genome_params = [single_genome_params, single_genome_params]
ea_params = {"n_offsprings": 4, "mutation_rate": 0.03, "n_processes": 1}
evolve_params = {"max_generations": int(args["--max-generations"]), "termination_fitness": 0.0}
We create a population that will be evolved
pop = cgp.Population(**population_params, genome_params=genome_params)
and an instance of the (mu + lambda) evolutionary algorithm
ea = cgp.ea.MuPlusLambda(**ea_params)
We define a callback for recording of fitness over generations
history = {}
history["fitness_champion"] = []
def recording_callback(pop):
history["fitness_champion"].append(pop.champion.fitness)
and finally perform the evolution
cgp.evolve(pop, objective, ea, **evolve_params, print_progress=True, callback=recording_callback)
Out:
[2/300] max fitness: -30.901735782503778[K
[3/300] max fitness: -30.901735782503778[K
[4/300] max fitness: -20.460618313162673[K
[5/300] max fitness: -20.460618313162673[K
[6/300] max fitness: -17.767232897765027[K
[7/300] max fitness: -17.767232897765027[K
[8/300] max fitness: -17.767232897765027[K
[9/300] max fitness: -17.767232897765027[K
[10/300] max fitness: -17.767232897765027[K
[11/300] max fitness: -17.767232897765027[K
[12/300] max fitness: -17.767232897765027[K
[13/300] max fitness: -17.767232897765027[K
[14/300] max fitness: -17.767232897765027[K
[15/300] max fitness: -17.767232897765027[K
[16/300] max fitness: -17.767232897765027[K
[17/300] max fitness: -17.767232897765027[K
[18/300] max fitness: -17.767232897765027[K
[19/300] max fitness: -17.767232897765027[K
[20/300] max fitness: -17.767232897765027[K
[21/300] max fitness: -17.767232897765027[K
[22/300] max fitness: -17.767232897765027[K
[23/300] max fitness: -17.767232897765027[K
[24/300] max fitness: -17.767232897765027[K
[25/300] max fitness: -17.767232897765027[K
[26/300] max fitness: -17.767232897765027[K
[27/300] max fitness: -17.767232897765027[K
[28/300] max fitness: -17.767232897765027[K
[29/300] max fitness: -17.767232897765027[K
[30/300] max fitness: -17.767232897765027[K
[31/300] max fitness: -17.767232897765027[K
[32/300] max fitness: -17.767232897765027[K
[33/300] max fitness: -17.767232897765027[K
[34/300] max fitness: -17.767232897765027[K
[35/300] max fitness: -17.767232897765027[K
[36/300] max fitness: -17.767232897765027[K
[37/300] max fitness: -17.767232897765027[K
[38/300] max fitness: -17.767232897765027[K
[39/300] max fitness: -17.767232897765027[K
[40/300] max fitness: -17.767232897765027[K
[41/300] max fitness: -17.767232897765027[K
[42/300] max fitness: -17.767232897765027[K
[43/300] max fitness: -17.767232897765027[K
[44/300] max fitness: -17.767232897765027[K
[45/300] max fitness: -8.357349504202604[K
[46/300] max fitness: -2.101173731642708[K
[47/300] max fitness: -2.101173731642708[K
[48/300] max fitness: -1.584095706383146[K
[49/300] max fitness: -1.584095706383146[K
[50/300] max fitness: -1.584095706383146[K
[51/300] max fitness: -1.584095706383146[K
[52/300] max fitness: -1.2705362372221112[K
[53/300] max fitness: -1.2705362372221112[K
[54/300] max fitness: -1.2705362372221112[K
[55/300] max fitness: -1.2705362372221112[K
[56/300] max fitness: -1.2705362372221112[K
[57/300] max fitness: -1.2705362372221112[K
[58/300] max fitness: -1.2705362372221112[K
[59/300] max fitness: -1.2705362372221112[K
[60/300] max fitness: -1.2705362372221112[K
[61/300] max fitness: -1.2705362372221112[K
[62/300] max fitness: -1.2705362372221112[K
[63/300] max fitness: -1.2705362372221112[K
[64/300] max fitness: -1.2705362372221112[K
[65/300] max fitness: -1.2705362372221112[K
[66/300] max fitness: -1.2705362372221112[K
[67/300] max fitness: -1.2705362372221112[K
[68/300] max fitness: -1.2705362372221112[K
[69/300] max fitness: -1.2705362372221112[K
[70/300] max fitness: -1.2705362372221112[K
[71/300] max fitness: -1.2705362372221112[K
[72/300] max fitness: -1.2705362372221112[K
[73/300] max fitness: -1.2705362372221112[K
[74/300] max fitness: -1.2705362372221112[K
[75/300] max fitness: -1.2705362372221112[K
[76/300] max fitness: -1.2705362372221112[K
[77/300] max fitness: -1.2705362372221112[K
[78/300] max fitness: -1.2705362372221112[K
[79/300] max fitness: -1.2705362372221112[K
[80/300] max fitness: -1.2705362372221112[K
[81/300] max fitness: -1.2705362372221112[K
[82/300] max fitness: -1.2705362372221112[K
[83/300] max fitness: -1.2705362372221112[K
[84/300] max fitness: -1.2705362372221112[K
[85/300] max fitness: -1.2705362372221112[K
[86/300] max fitness: -1.2705362372221112[K
[87/300] max fitness: -1.2705362372221112[K
[88/300] max fitness: -1.2705362372221112[K
[89/300] max fitness: -1.2705362372221112[K
[90/300] max fitness: -1.2705362372221112[K
[91/300] max fitness: -1.2705362372221112[K
[92/300] max fitness: -1.2705362372221112[K
[93/300] max fitness: -1.2705362372221112[K
[94/300] max fitness: -1.2705362372221112[K
[95/300] max fitness: -1.2705362372221112[K
[96/300] max fitness: -1.2705362372221112[K
[97/300] max fitness: -1.2705362372221112[K
[98/300] max fitness: -1.2705362372221112[K
[99/300] max fitness: -1.2705362372221112[K
[100/300] max fitness: -1.2705362372221112[K
[101/300] max fitness: -1.2705362372221112[K
[102/300] max fitness: -1.2705362372221112[K
[103/300] max fitness: -1.2705362372221112[K
[104/300] max fitness: -1.2705362372221112[K
[105/300] max fitness: -1.2705362372221112[K
[106/300] max fitness: 0.0[K
After finishing the evolution, we print the evolved expression and plot the result.
expr = pop.champion.to_sympy()
print(expr)
print(f"--> x<=0: {expr[0]}, \n x> 0: {expr[1]}")
width = 9.0
fig, axes = plt.subplots(1, 2, figsize=(width, width / scipy.constants.golden))
ax_fitness, ax_function = axes[0], axes[1]
ax_fitness.set_xlabel("Generation")
ax_fitness.set_ylabel("Fitness")
ax_fitness.plot(history["fitness_champion"], label="Champion")
ax_fitness.set_yscale("symlog")
ax_fitness.set_ylim(-1.0e2, 0.1)
ax_fitness.axhline(0.0, color="0.7")
f = pop.champion.to_numpy()
x = np.linspace(-5.0, 5.0, 20)[:, np.newaxis]
y = np.piecewise(x, [x < 0, x >= 0], f)
y_target = f_target(x)
ax_function.plot(x, y_target, lw=2, alpha=0.5, label="Target")
ax_function.plot(x, y, "x", label="Champion")
ax_function.legend()
ax_function.set_ylabel(r"$f(x)$")
ax_function.set_xlabel(r"$x$")
fig.savefig("example_multi_genome.pdf", dpi=300)
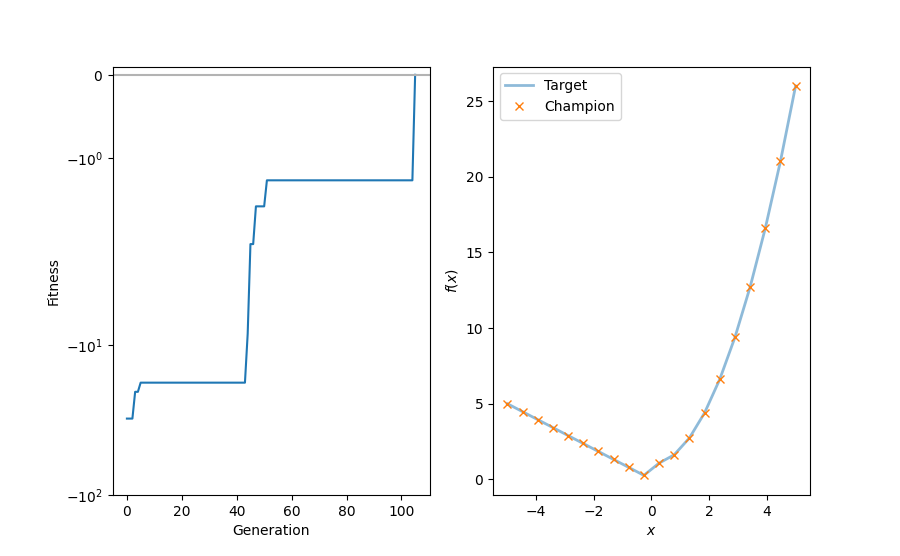
Out:
[-x_0, x_0**2 + 1.0]
--> x<=0: -x_0,
x> 0: x_0**2 + 1.0
Total running time of the script: ( 0 minutes 0.919 seconds)